Other
-
Daniel Gurevich
-
Princeton University
-
Data-driven inference of symmetry-equivariant models of natural phenomena
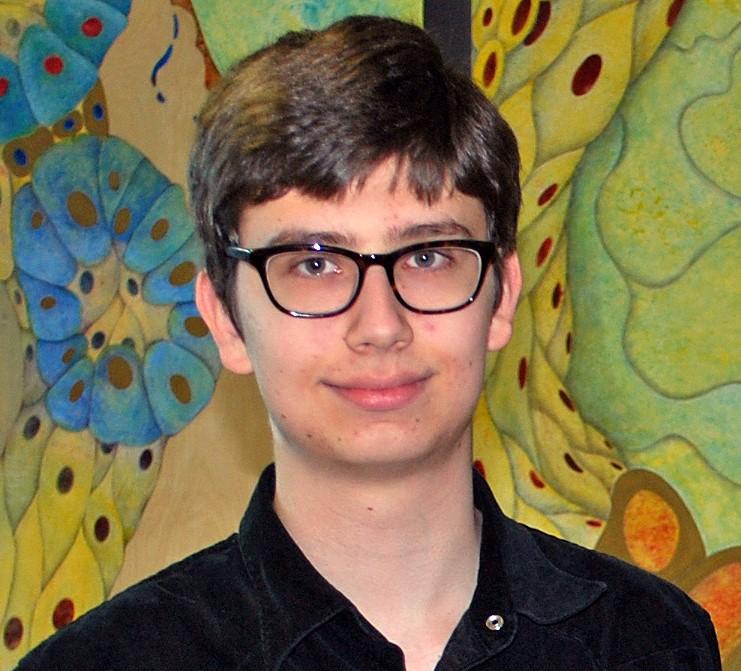
Data-driven inference of symmetry-equivariant models of natural phenomena
Advisors: Charles Fefferman and Clarence Rowley
Abstract:
This dissertation provides an in-depth description of the SPIDER (Sparse Physics- Informed Discovery of Empirical Relations) framework for data-driven inference of symmetry-equivariant models of spatiotemporally extended physical systems. The homogeneity and isotropy of physical space require mathematical models of natural phenomena to be equivariant with respect to symmetries including translations, rotations, and reflections. Unstructured data-driven model inference leads to an in- tractable optimization problem due to the high dimensionality of both the spatiotemporal data and the model search space. In contrast, SPIDER exploits tensor representations of the symmetry group, alongside other physical constraints such as local or short-range interactions, to describe systems via sets of tensor-valued partial differential or integro-differential equations, which can parsimoniously and interpretably parametrize a wide range of models.
While prior work touches on necessary ingredients for the successful application of SPIDER to complex real-world problems, such as weak formulation of differential equations and sparse regression, this manuscript addresses essential gaps that have precluded more widespread adoption. Namely, it (1) formalizes an algorithm for automatically generating and manipulating libraries of symmetry-equivariant tensors, (2) describes how to synthesize all logically independent equations in the search space, and (3) generalizes SPIDER to discover mean-field models of many-body systems composed of discrete particles or agents. These innovations are implemented as part of a software package, PySPIDER, and illustrated using two examples representing an incompressible fluid flow in the continuum limit and a compressible fluid consisting of discrete interacting particles.