-
Spectral barriers in random certification problems
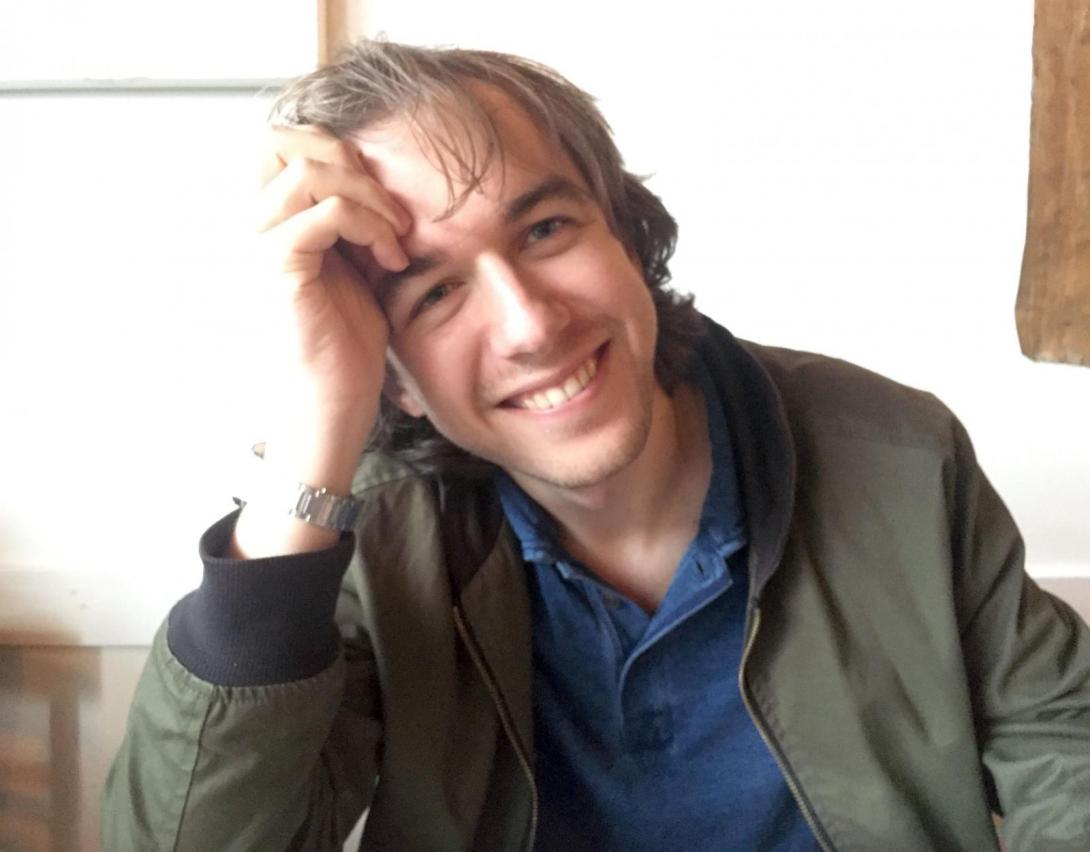
Spectral barriers in random certification problems
Certifying bounds on optimization problems---bounding the best-possible solution rather than merely producing a single good solution---is an important class of algorithmic tasks ubiquitous in combinatorial and continuous optimization, and especially intimately related to convex relaxation techniques. I will present theoretical evidence that, for a class of random problems including max-cut, graph coloring, and various structured PCA problems, there is a fundamental obstruction to efficiently certifying bounds better than a naive spectral bound. Consequently, even sophisticated linear and semidefinite programming relaxations perform no better on average than a simple spectral relaxation. I will show how this conclusion follows from the possibility of "planting" high-quality solutions in a problem instance in a way that is "quiet" or difficult to detect. Time permitting, I will also discuss how this quietness may be predicted with hypothesis testing algorithms based on low-degree polynomials, as well as how this framework suggests a new approach to lower bounds in the sum-of-squares semidefinite programming hierarchy, as well as joint work with Afonso Bandeira and Alex Wein.
Tim Kunisky is a fifth-year PhD student in the Courant Institute of Mathematical Sciences at New York University, advised by Afonso Bandeira and Gérard Ben Arous. His current research interests include: Computational "hard regimes" in problems arising in data science and optimization; convex relaxations and their average-case performance on random problems; and random optimization landscapes and the relationship between their high-dimensional geometry and the computational hardness of optimization.