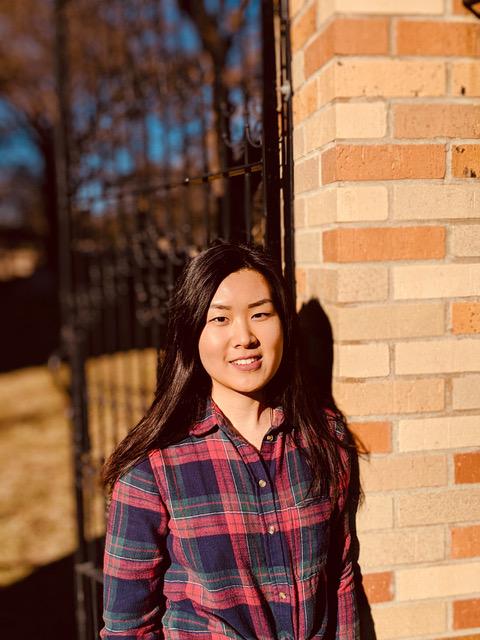
Zoom: click here
Meeting ID: 914 5103 5364 Passcode: 600983
Tensor-structured dimensionality reduction and applications
Abstract: Many scientific and engineering applications utilize a tensor format for data representations. However, memory and computational costs can be prohibitive due to the exponentially growing size of higher-order tensors. To address these barriers, we focus on both computational and theoretical aspects of tensor dimensionality reduction methods. We adopt classical reduction approaches such as random projections and principal component analysis (PCA), and pursue scalable algorithms tailored to solving tensor programs. We also discuss theoretical accuracy guarantees for reduced data.
Ruhui Jin is a mathematics PhD candidate from the University of Texas at Austin, advised by Rachel Ward. Her primary research is on mathematical foundations of data science. She has been applying techniques from numerical multi-linear algebra, applied probability and optimization theory to solve problems involving high-dimensional datasets. She received a BSc (Hons) in mathematics from Sichuan University, Chengdu, China in 2017.