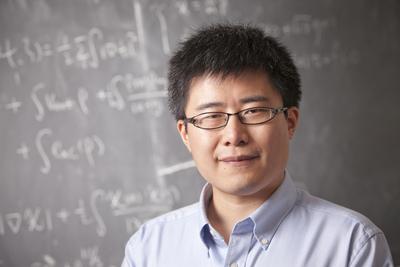
Prof. Jianfeng Lu
Analysis of score-based generative models
Abstract: Score-based generative modeling (SGM) is a highly successful approach for learning a probability distribution from data and generating further samples, based on learning the score function (gradient of log-pdf) and then using it to simulate a stochastic differential equation that transforms white noise into the data distribution.
In this talk, we will discuss some recent works in convergence analysis of SGM. In particular, we established convergence of SGM applying to any distribution with bounded 2nd moment, relying only on a $L^2$-accurate score estimate, with polynomial dependence on all parameters and no reliance on smoothness or functional inequalities.
Based on joint works with Hongrui Chen (Peking University), Holden Lee (Johns Hopkins) and Yixin Tan (Duke).
Bio: Jianfeng Lu is a Professor of Mathematics, Physics, and Chemistry at Duke University. Before joining Duke University, he obtained his PhD in Applied Mathematics from Princeton University in 2009 and was a Courant Instructor at New York University from 2009 to 2012. He works on mathematical analysis and algorithm development for problems and challenges arising from computational physics, theoretical chemistry, materials science, high-dimensional PDEs, and machine learning. His work has been recognized by a Sloan Fellowship, a NSF Career Award, and the 2017 IMA Prize in Mathematics and its Applications.