-
Diffusion operators for multi-modal data analysis
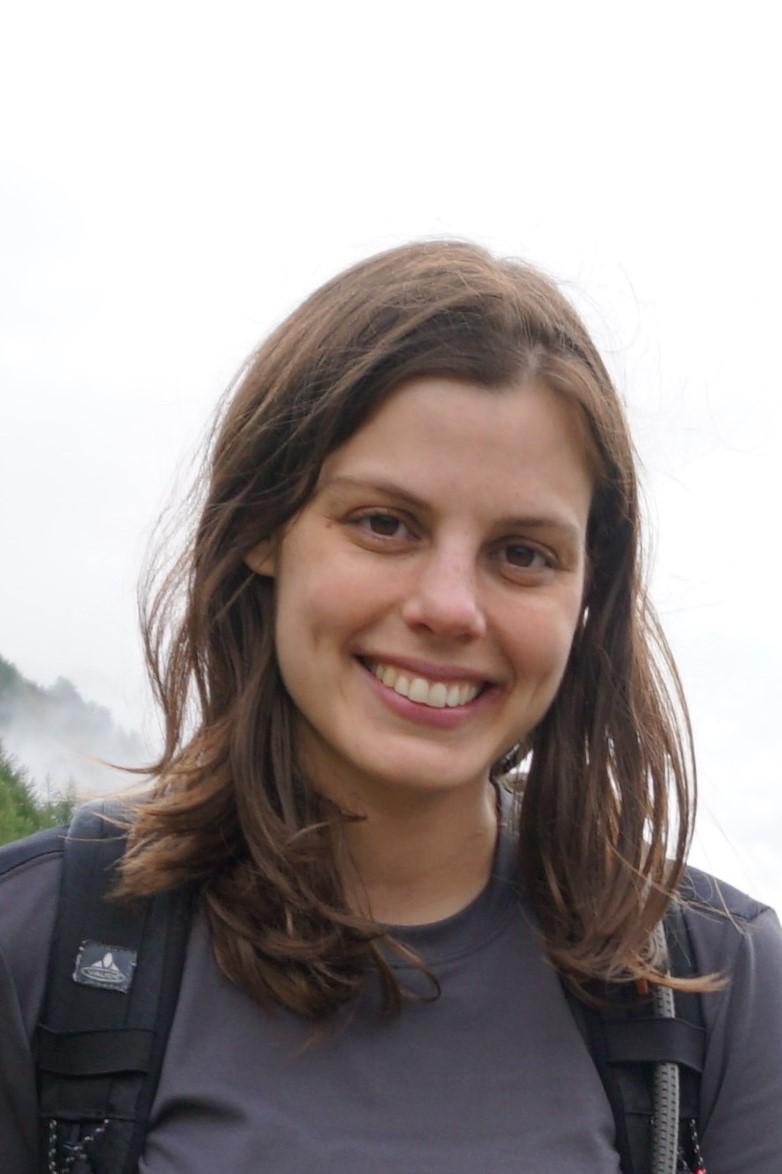
Diffusion operators for multi-modal data analysis
Finding appropriate low dimensional representations of high-dimensional multi-modal data can be challenging since each modality embodies unique deformations and interferences. In this talk, I will present our recent work, which addresses the problem using manifold learning, where the data from each modality is assumed to lie on some manifold. In this context, the goal is to characterize the relations between the different modalities by studying their underlying manifolds. Two new operators are proposed allowing to isolate, enhance and attenuate the hidden components of multi-modal data in a data-driven manner. Based on these new operators, efficient low-dimensional representations can be constructed for such data, which characterize the common structures and the differences between the manifolds underlying the different modalities. The capabilities of the proposed operators are demonstrated on several applications.
Tal Shnitzer is a Ph.D. student in the Electrical Engineering faculty at the Technion - Israel Institute of Technology, under the supervision of Prof. Ronen Talmon. She completed her B.Sc in Biomedical Engineering and Electrical Engineering in 2013 at the Technion. Her research focuses on developing algorithms based on geometric modeling methods for time series analysis and sensor fusion.