-
Learning from noise: data-driven processing of low SNR images with application to cryo-electron microscopy
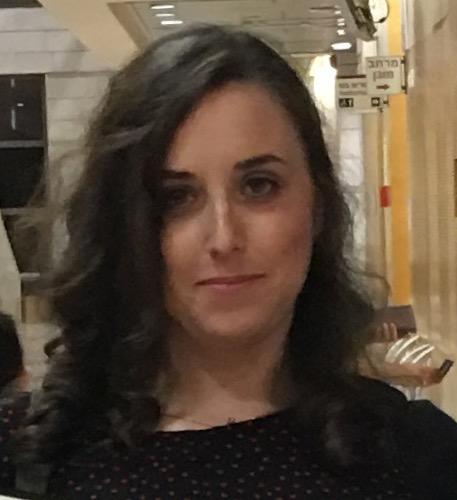
Learning from noise: data-driven processing of low SNR images with application to cryo-electron microscopy
Single-particle cryo-electron microscopy aims to determine the structure of 3D macromolecules from multiple 2D projections. The high levels of noise present in experimental data, in conjunction with a distortion applied by the electron microscope, is a cause of significant challenges to the process of acquiring particle stacks from the raw data outputted by the electron microscope. First and foremost, the noise and distortion complicate the selection of particle projections, making edge detection methods ineffective and adding many pitfalls to template-based methods. Furthermore, even after successful particle picking, any 3D volume reconstructed from the distorted projections selected may yield an unreliable representation of the macromolecule. It is, therefore, necessary to estimate the distortion applied by the electron-microscope to each of the picked projection images.
In this talk, I will introduce a simple and novel approach for fast, accurate, and template-free particle picking. This method, which can be considered as a mirror-image of template matching, includes no model for the particle and instead focuses on prior knowledge on the noise. I will also discuss a novel method for estimating the distortion applied by the electron-microscope and include an evaluation of our methods on several publicly available datasets.
Ayelet Heimowitz is a postdoctoral Research Associate at the Program in Applied and Computational Mathematics at Princeton University. She received her Ph.D. from the Faculty of engineering at Bar Ilan University in 2016. Her research focuses on developing algorithms for analysis of noisy, massive and partial data.